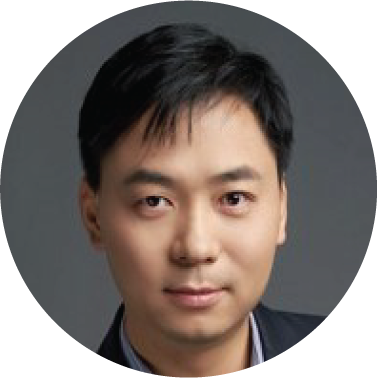
Professor Tao Da Cheng
Dacheng Tao is currently a Professor of Computer Science, Peter Nicol Russell Chair and an Australian Laureate Fellow in the Sydney AI Centre and the School of Computer Science in the Faculty of Engineering at The University of Sydney. He mainly applies statistics and mathematics to artificial intelligence and data science, and his research is detailed in one monograph and over 200 publications in prestigious journals and proceedings at leading conferences. He received the 2015 and 2020 Australian Eureka Prize, the 2018 IEEE ICDM Research Contributions Award, and the 2021 IEEE Computer Society McCluskey Technical Achievement Award. He is a Fellow of the Australian Academy of Science, AAAS, ACM and IEEE.
Abstract: Human Pose Detection for Lifespan Healthcare
Vision-based human pose detection plays a crucial role in enhancing lifespan healthcare, particularly in remote monitoring, posture analysis and rehabilitation. However, it presents significant challenges such as high motion variability, occlusions, and limited training data. While traditional probabilistic graphical models struggled to address these complexities, Convolutional Neural Networks (CNNs) have shown promise in improving accuracy. Nevertheless, CNNs’ limitations, including misdetections in crowded scenes and significant jitter in static scenarios, hinder their application in healthcare. Fortunately, the emergence of vision foundation models has brought about a transformative change in this field. Embracing the philosophy of “More is Different, Greatness in Simplicity,” we introduce ViTPose, a robust system that harnesses vision foundation models for automated pose detection from everyday videos. By incorporating attention operations, ViTPose effectively extracts both local and global information, thus enhancing robustness against challenging scenarios. This paradigm shift has democratized access to accurate pose detection and expanded its applications in healthcare, most notably by enabling precise quantitative motor assessments. These advancements bring us closer to realizing the full potential of vision-based human pose detection in lifespan healthcare. While further refinement and maturation are still required, the profound impact of deep learning driven by foundation models, or super deep learning, in revolutionizing this field cannot be overstated. Super deep learning holds the promise of delivering increasingly sophisticated and robust pose detection solutions, thereby shaping the future of healthcare and significantly enhancing the quality of patient care throughout their lifespan.